Table of Contents
- Deep learning dominates AI, but needs an update to maintain its hegemony and take the field to the next level
- Eliminate Convolutional Neural Networks
- Self-supervised deep learning
- Hybrid Model: Symbolic AI + Deep Learning
- Deep learning for system 2
- Deep learning based on neuroscience
- Conclusion
Deep learning dominates AI, but needs an update to maintain its hegemony and take the field to the next level
Humans are an inventing race. The world provides us with raw materials, which we transform with skillful techniques. Technology has created countless tools and devices. The wheel, the printing press, the steam engine, the automobile, electricity, the Internet…these inventions shaped our civilization and culture, and still do.
One of the latest technological babies we’ve created is artificial intelligence, which has become an integral part of our lives in recent years. Its impact on society is striking and is expected to continue to grow over the coming decades . One of AI’s leading faces, Andrew Ng, even said, ” AI is the new electricity. ” In an interview with Stanford Business magazine, he said, “Just as electricity changed almost everything 100 years ago, today it’s really hard to think of an industry that AI won’t change in the next few years.” (*translation note 1).
But AI is nothing new. It has been around since John McCarthy coined the term AI in 1956 and proposed it as his own field of research. Since then, AI has alternated between periods of indifference and constant funding and interest . Machine learning and deep learning (hereinafter “DL” stands for deep learning) currently dominate AI. The DL revolution that began in 2012 isn’t over yet. DL has the AI crown, but experts say a few changes are needed to maintain that crown. Let’s take a look at the future of DL below.
In an interview with Andrew Ng published by Stanford Business magazine in March 2017, he argued that AI is the new electricity, as well as:
- Lack of human resources and lack of data are delaying the social implementation of AI .
- Concerns about the birth of “evil AI” or “killer robots” are as nonsense as worrying about overpopulation on Mars .
- The US government should reform the education system and develop safety nets in order to respond to the restructuring of the working environment by AI .
[ [Understand in 5 minutes] AI research, complete explanation of 60 years of history! ]
“ [For qualification measures! ] Review the history of AI
from the Dartmouth Conference to the Singularity chronologically ”
・・・
Eliminate Convolutional Neural Networks
DL’s popularity skyrocketed when Jeffrey Hinton’s team, dubbed the “Godfather of AI,” won the 2012 ImageNet challenge with a model based on convolutional neural networks ( CNNs). They achieved a top-1 accuracy of 63.30%, wiping out their (non-DL) rivals with an error of more than 10%. The success and interest that DL has generated over the last decade can be attributed to CNN.
CNN-based models are very popular for computer vision tasks such as image classification , object detection , and face recognition . But despite its usefulness, Hinton pointed out one key drawback in his AAAI 2020 keynote . “[CNN] isn’t very good at dealing with the effects of perspective changes like rotation and scaling,” he said.
CNNs can handle transforms (of images). However, while the human visual system can recognize objects under different viewing angles, backgrounds, and lighting conditions, CNNs cannot. The current best CNN system, which achieves over 90% top-1 accuracy on the ImageNet benchmark, suffers a 40-45 % performance degradation when trying to classify images of real-world object datasets. translation note 3).
Another problem is the so-called adversarial case . Hinton reiterates the differences between the human visual system and CNNs. “If you add a little bit of noise to an image, the CNN perceives it as something completely different, but I can hardly tell that it has changed. I think it’s evidence that we’re recognizing images using completely different information.” CNNs are fundamentally different from the human visual system. You can’t rely on CNN simply because it’s unpredictable.
Hinton goes one step further by explaining that CNN systems cannot interpret objects in images. We know that objects exist in the world, and we have experience with them. From a very early age , I know about solidity, shape constancy, or the permanence of objects. We can use this knowledge to make sense of strange objects, but CNNs only see bundles of pixels. We may need to fundamentally shift the computer vision paradigm, and that shift may be capsule networks . The father of quantum mechanics, Max Planck, said:
“Science advances with each funeral”
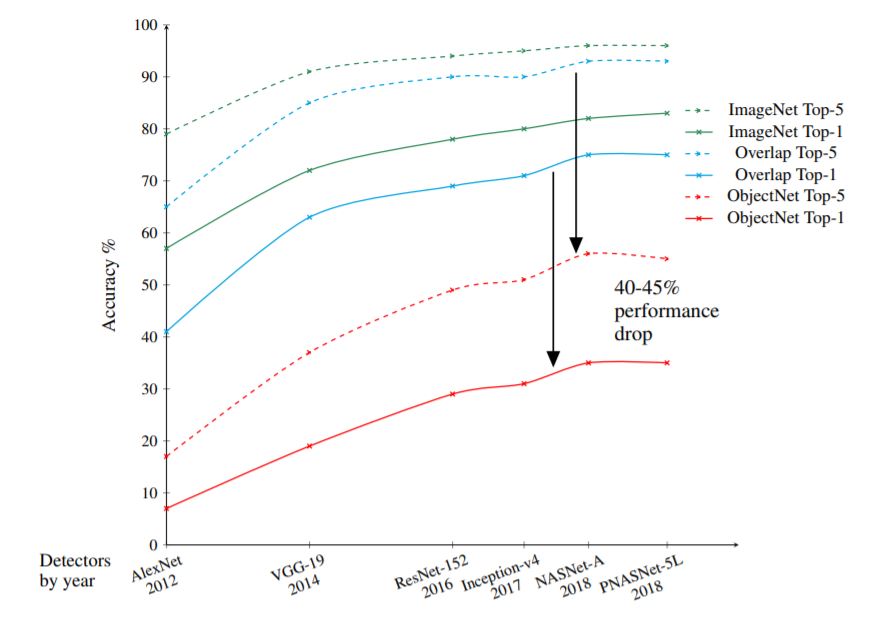
Image source: Graph from the paper ” ObjectNet: A large-scale bias-controlled dataset for pushing the limits of object recognition models “
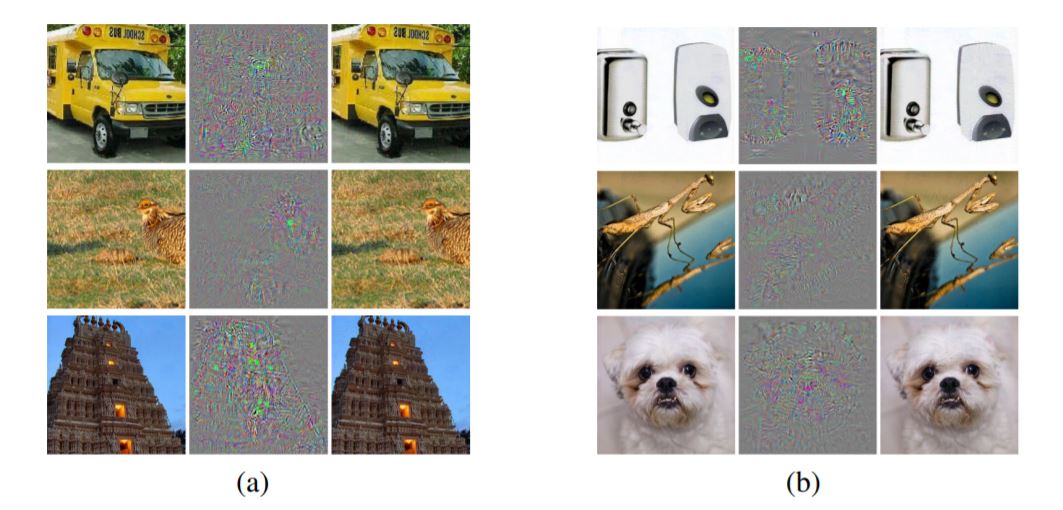
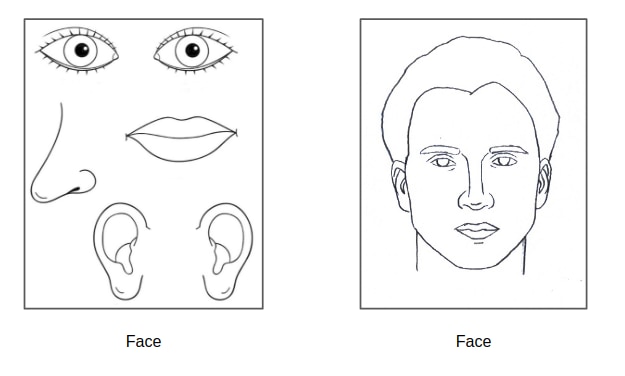
Self-supervised deep learning
“The next revolution in AI will be neither supervised nor reinforcement learning at all”
– Yann LeCun, Chief AI Scientist at Facebook
One of the current limitations of DL is its reliance on huge amounts of labeled data and computing power. DL pioneer Yann LeCun says we need to replace supervised learning, the way most DL systems learn, with what he calls ” self-supervised learning .”
(Self-supervised learning) is the idea of learning to represent the world before learning a task. This is what infants and animals do. Once the world is well represented, fewer trials and fewer samples are required to learn the task.
Instead of training the system on labeled data, the system learns labels from raw data. We humans learn orders of magnitude faster than supervised (or reinforcement learning) systems. Children don’t learn to recognize trees by looking at hundreds of images of trees. Look at a single image and label what you intuitively know belongs to that category as “tree”. We may learn by observation , which computers cannot yet do.
Yann LeCun gave an in -depth talk on this topic in December 2019 . He argued that a self-supervised system would be able to “predict any part of the input from any other part.” For example, it can predict the future from the past, or the masked part from the visible part. However, while this kind of learning is effective for discrete inputs such as text ( Google’s BERT and OpenAI’s GPT-3 are good examples), it is less effective for continuous data such as images, audio, and video. ineffective. He explained that this requires a latent-variable energy-based model suitable for dealing with the uncertainty inherent in the world .
Self-supervised learning will drive out supervised learning. There are still issues to be solved, but the bridge to bridge the gap has already begun. Once you get into the world of self-supervised learning, it’s clear that there’s no going back.
“Labels are the opium of machine learning researchers”
– Jitendra Malik, Professor of Electrical Engineering & Computer Science, University of California, Berkeley
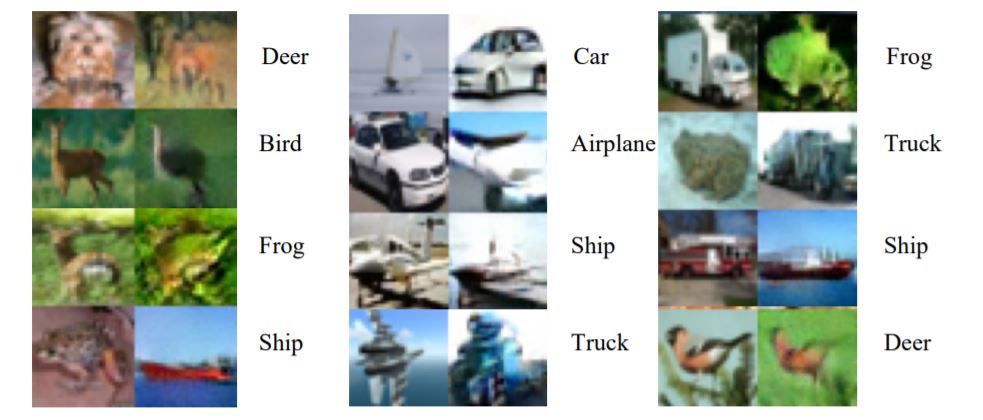
Image source: Image from the paper ” Implicit Generation and Modeling with Energy-Based Models “
・・・
Hybrid Model: Symbolic AI + Deep Learning
Two paradigms, symbolic AI (aka rule-based AI) and DL, have been overwhelmingly popular since the dawn of AI. Symbolic AI was all the rage from the 50s to the 80s, but most experts now disagree with the framework. John Haugeland calls it “GOFAI” (Good Old-Fashioned Artificial Intelligence) in his book Artificial Intelligence: The Great Idea .
(Symbolic AI) deals with abstract representations of the real world modeled in an expression language based primarily on mathematical logic.
Symbolic AI is top-down AI. This is based on the “ Physical Symbolic System Hypothesis ‘ ‘ advocated by Allen Newell and Herbert Simon . It is. Expert systems , representatives of this class of AI, for example, are designed to emulate human decision-making based on if-then rules.
The hybrid model is an attempt to combine the strengths of symbolic AI and DL. In his book The Architect of Intelligence, Martin Ford interviews AI experts about this approach. Andrew Ng emphasizes its usefulness when tackling problems with small datasets. Josh Tenenbaum, Professor of Computational Cognitive Sciences at MIT, worked with his team to develop a hybrid model that “learns the semantic analysis of visual concepts, words, and sentences all without explicit supervision.” did.
Gary Marcus, a professor of psychology at New York University, argues that common sense reasoning is better off with a hybrid model. In his recent paper , he cites human intelligence to underscore his point.
Manipulating symbols in some way seems essential to human cognition, such as when a child learns the meaning of a word that can be applied to countless family members, such as “little sister.”
Despite its promise, the hybrid approach has serious opponents. Jeffrey Hinton criticizes those who intend to mess with DL with symbolic AI. “Hybrid model advocates must admit that deep learning is doing amazing things, but they are like low-level servants providing what is needed to make symbolic reasoning work. As a thing, we’re going to use deep learning,” he said. That said, success or failure aside, hybrid models will be something to keep an eye on for years to come.
“In a few years, many will wonder why deep learning has been trying to achieve such great results for so long without the wonderful and valuable tool of symbolic manipulation. Deaf and I predict.”
– Gary Marcus
・・・
Deep learning for system 2
Yoshua Bengio, part of the 2018 Turing Award winner trio (with Hinton and Lucan), gave a talk in 2019 titled ” From Deep Learning in System 1 to Deep Learning in System 2. ” He talked about the current state of DL where the trend is to make everything bigger: bigger datasets, bigger computers, bigger neural nets. He argued that this direction would not lead to the next stage of AI.
“We have machines that learn very narrowly. They require much more data than the training case of human intelligence, and [yet] they make stupid mistakes.”
Bengio adopts the framework of two systems advocated by Daniel Kahneman in his book Thinking , Fast & Slow . is doing. Kahneman describes System 1 as “automatic and quick to act with little or no effort, without a sense of voluntary control,” whereas System 2 “often is subject to agency, choice, and control.” , associated with subjective experiences such as concentration and attention to mental activities that require effort.”
Rob Towes summarizes the current state of DL as follows: “Current state-of-the-art AI systems are great at System 1 tasks, but struggle very hard at System 2 tasks.” Bengio is of the same opinion. “We [humans] can come up with algorithms and recipes, plan, reason, and use logic. I hope that the future of deep learning will be able to handle these issues as well.”
Bengio argues that System 2’s DL will be able to generalize to “differently distributed data”, so-called out-of-order distributions . Currently, DL systems need to be trained and tested on the same distributed dataset, and this need corresponds to the hypothesis of independent and identically distributed data. “We need a system that can continuously learn on heterogeneous data,” he said. System 2 DL will succeed using non-uniform real-world data.
This will require systems with better transfer learning capabilities. Bengio suggests that attention mechanisms and meta-learning (learning to learn) are fundamental building blocks in System 2 cognition . To underscore the importance of learning to adapt to the ever-changing world demanded of System 2 AI, let me cite a passage expressing a central idea in Darwin’s masterpiece On the Origin of Species ( he said: (*Translation Note 10)) .
“It is not the strongest species that survives, nor the most intelligent, but the most adaptable.”
A case in which IID is observed is the roll of the dice. If a die rolls a “6” 20 times in a row, the probability of rolling a “6” on the 21st roll is independent and identical to the previous rolls, with a “1 in 6” probability. IID is a premise of cross-validation that evaluates performance by dividing the data collected when building an AI model into learning data and test data .
・・・
Deep learning based on neuroscience
“Artificial Neural Networks are just rough representations of how the brain works.”
– David Sucilo, Google Brain Group
The decade of 1950 saw several important scientific breakthroughs that laid the groundwork for the birth of AI. Neuroscience studies have found that the brain is made up of neural networks that “fire with all-or-nothing pulses.” This discovery, combined with theoretical descriptions such as cybernetics , information theory , and Alan Turing’s theory of computation , suggested the possibility of creating an artificial brain.
AI originated in the human brain, but today’s DL doesn’t work like the human brain . The differences between DL systems and the human brain have already been subtly touched upon in this article. CNNs don’t work like the human visual system. We humans observe the world rather than learn from labeled data. It also combines bottom-up processing with top-down symbolic manipulation. Then, it recognizes System 2. The ultimate goal of AI was to build an electronic brain that could simulate our brain, an artificial general intelligence (some would call it strong AI ). Neuroscience can help DL toward this goal.
Neuromorphic computing, which stands for hardware that simulates the structure of the brain, is one important approach. I wrote in a previous article that there is a big difference between biological neural nets and artificial neural nets. “Neurons in the brain transmit information in the timing and frequency of spikes, keeping the signal strength (voltage) constant. Artificial neurons are the opposite. Attempts to convey information only with the strength of Neuromorphic computing seeks to reduce this difference.
Another drawback of artificial neurons is their simplicity. Artificial neurons are built on the premise that biological neurons are ” bad calculators that do only basic calculations .” But this premise is far from the truth. In a study published in the journal Science , a group of German researchers said , “Single neurons may indeed be able to perform complex functions, such as recognizing objects by themselves. ‘, he showed.
“Maybe there is a deep network in one neuron (in the brain)”
– Joita Poiraj, Institute of Molecular Biology & Biotechnology (Hellas Research and Technology Foundation, Greece)
In a paper published in Neuron , DeepMind CEO and co-founder Dennis Hassabis expressed the importance of using neuroscience to advance AI. Apart from some of the ideas mentioned above, his paper highlights two important aspects. Intuitive physics and planning.
James R. Kublich and his colleagues define intuitive physics as “the ability to understand the physical environment, interact with objects and matter that dynamically change states, and learn how observed events evolve. knowledge underlying the human ability to predict at least approximately The DL system has no such knowledge. This is because DL systems do not exist in the world, are not embodied, and do not carry the evolutionary baggage that has helped humans adapt to their surroundings. . Josh Tenenbaum is working on imbuing machines with this ability .
Planning can be understood as “searching to decide what actions should be taken in order to achieve a given goal”. We do this on a daily basis, but the real world is too complicated for machines. DeepMind’s MuZero can play some world-famous games through planning, but these games have perfectly defined rules and boundaries.
The famous coffee test proposes a test in which an AI with planning ability walks into the average house, goes to the kitchen, fetches ingredients, and sees if it can make coffee. Planning requires the decomposition of complex tasks into subtasks, a capability that exceeds the capabilities of current DL systems. Yann LeCun admits that he “doesn’t know how to solve” the test.
There are many ideas that DL can get from neuroscience. If we’re going to get close to intelligence, what are we going to do without examining the only sample of intelligence we have, the human brain? Demis Hassabis says:
“With artificial intelligence having so many problems, the need for the fields of neuroscience and AI to come together is greater than ever.”
In the blog post ” What is the difference between artificial neural networks and the human brain? “, Verzeo, a startup that provides an AI-based online learning platform, describes the differences between the human brain and neural networks as follows: summarized in a table like this.
human brain | artificial neural network | |
size | 86 billion nerve cells | 10 to 1,000 neurons |
learning function | Vague concepts can also be learned | Learning ambiguous concepts requires precise and structured data |
topology | Complex topologies with asynchronous connections | layered tree-like topology |
energy consumption | Consume less energy than output. about 20 watts | Energy consumption greater than output |
・・・
Conclusion
The DL system is very convenient. Over the last few years, the DL system has single-handedly changed the tech landscape. However, in order to realize intelligent machines in the true sense of the word, it is necessary to abandon the notion that “bigger is better” and qualitatively innovate the DL system.
Several approaches exist today to achieve this milestone. Eliminating CNNs and their limitations, eliminating labeled data, combining bottom-up and top-down processing, implementing System 2 cognitive functions in machines, and incorporating ideas and advances from neuroscience and the human brain.
We do not know which path is best for realizing a truly intelligent system. In the words of Yann LeCun, ” no one has the perfect answer .” But I have high hopes that one day we’ll find the perfect answer.