Table of Contents
- What are the practical but less common ways to build AI skills when external talent is scarce or expensive?
- 5 Skills You Need to Leverage AI
- 1. Find people outside the IT team
- 2. Use publicly available content to tailor your data science curriculum
- 3. Bridge your team’s technical skills and domain expertise
- 4. Allow experimentation and learning at work
- Skill balance in the team
What are the practical but less common ways to build AI skills when external talent is scarce or expensive?
Analytics leaders at US-based Fortune 200 companies were under intense pressure. Her team supported 45,000 employees at a global energy company, but business users weren’t satisfied. Analytics deliverables were often delayed and had quality issues.
The analytics team was part of the IT organization and was struggling to fill vacancies. The required skills were not found within the IT team. Their offices were about 60 miles (97 kilometers) by car north of any major American city, so it wasn’t easy to recruit people.
Developing the few people they managed to hire wasn’t easy, and they often lacked business understanding. As a result, analytics teams have become notorious for being understaffed, overworked, and exposed to the ire of business users.
Doesn’t this story sound familiar to you?
Recruiting data science talent is one of the biggest challenges facing companies today. According to O’Reilly’s 2021 Artificial Intelligence (AI) Adoption Survey in Enterprises , the most frequently reported challenge is a lack of skilled personnel or difficulty in hiring the required positions. was done. As investment in AI increases across organizations, the competition for AI talent is heating up.
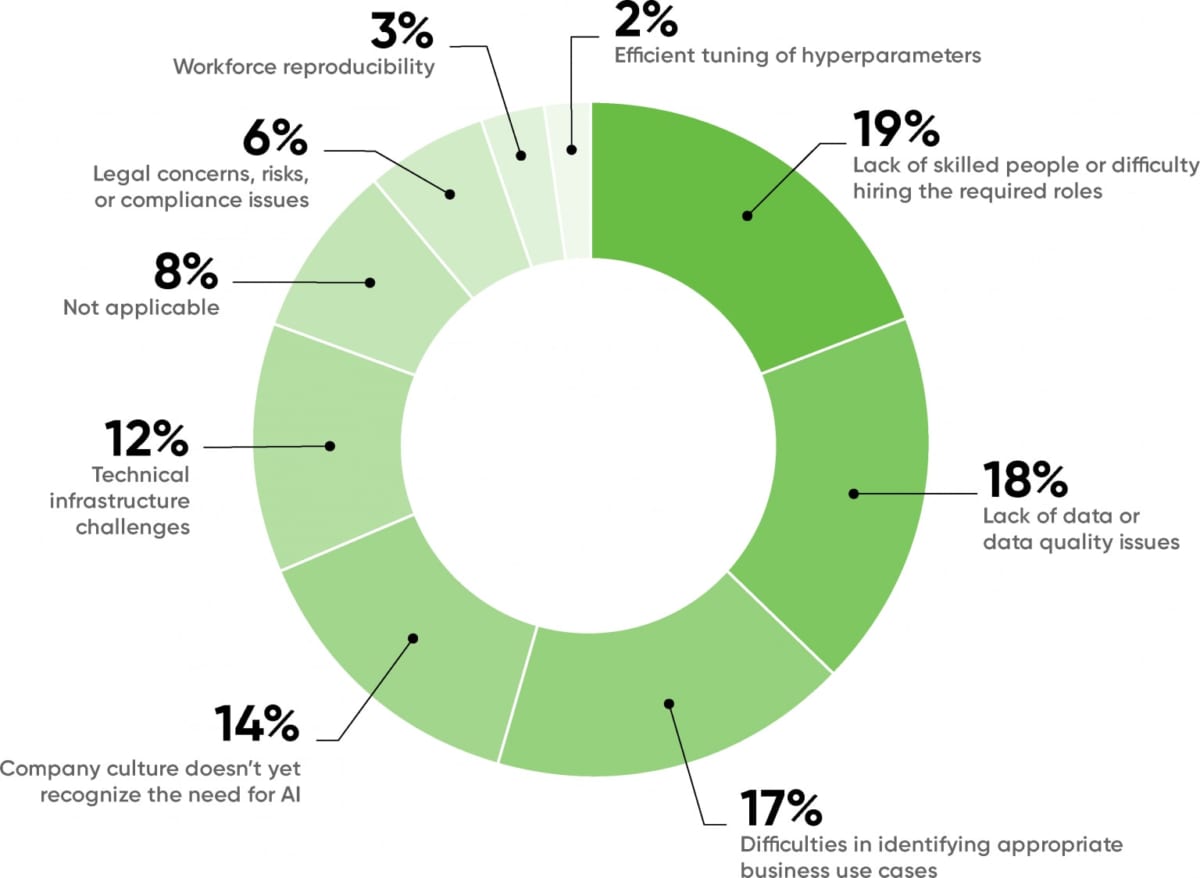
AI adoption bottleneck
5 Skills You Need to Leverage AI
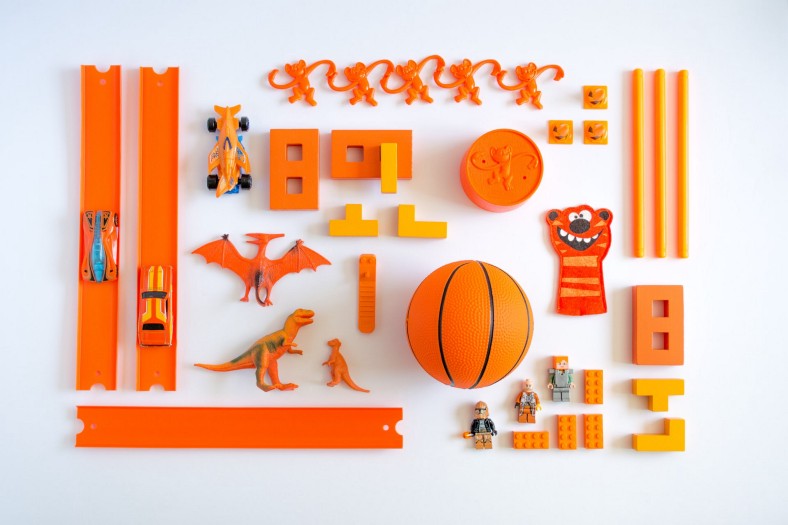
Image credit: Vanessa Bucceri on Unsplash
It is a misconception that building AI solutions requires only data scientists. AI is only possible with data. We need to collect, manage and store quality data. Once you have your data, you need five data science skills to successfully design, build, and deploy AI:
- Domain expertise: skills to pick the right business problem and build the right approach
- Machine Learning (ML): Skills for Identifying Data Insights and Building AI Models
- Software Engineering: Skills in packaging models as software applications
- Information Design: The skill of designing workflows and making model insights available to users
- Managerial Expertise: Skills to manage data project uncertainty and ensure user adoption
Here are four less common ways to develop these multifunctional skills in-house when external talent is scarce or expensive.
- Identify operations that can be improved with the introduction of AI .
- After identifying the work to be introduced with AI, a prototype is produced and the effect of introducing AI is evaluated .
- After confirming the effect of AI introduction , calculate the ROI (return on investment) of AI introduction and convince the executives. In addition, make it known that even if AI is introduced , humans will still be involved in the workflow .
1. Find people outside the IT team
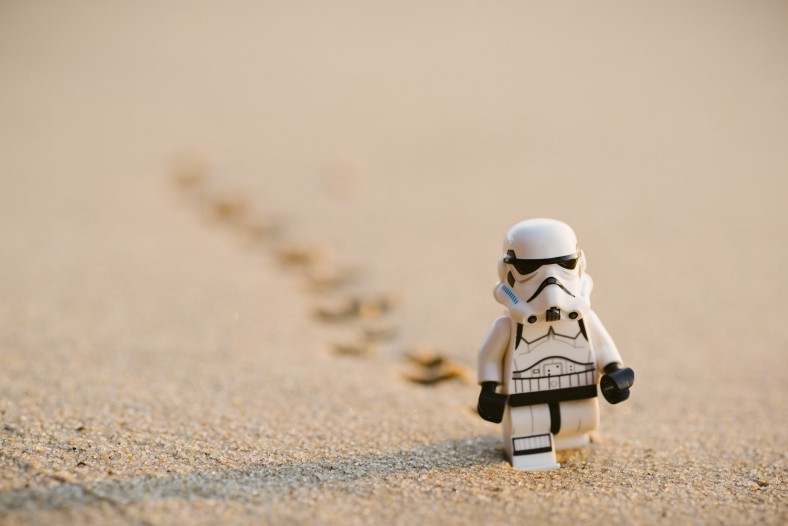
Image Source: Daniel Cheung on Unsplash
Lisa Palmer, Chief Technical Advisor at Splunk , said, “Every organization is underutilizing their current staff due to lack of awareness.” IT teams often limit their in-house talent searches to technical teams. “But you’d be surprised at how versatile and deep people are in business departments outside of IT,” she said.
To discover hidden talent in your organization, you must start by maintaining a list of skills that each employee claims to be. This list should be updated semi-annually, openly searchable by colleagues, and easy to use and useful. Palmer recommends classifying each individual’s skills into four categories: expert, functional, novice, and subject to improvement. These efforts will enable teams with hiring needs to scout individuals who are skill-ready and willing to grow on the five competencies required for AI.
2. Use publicly available content to tailor your data science curriculum
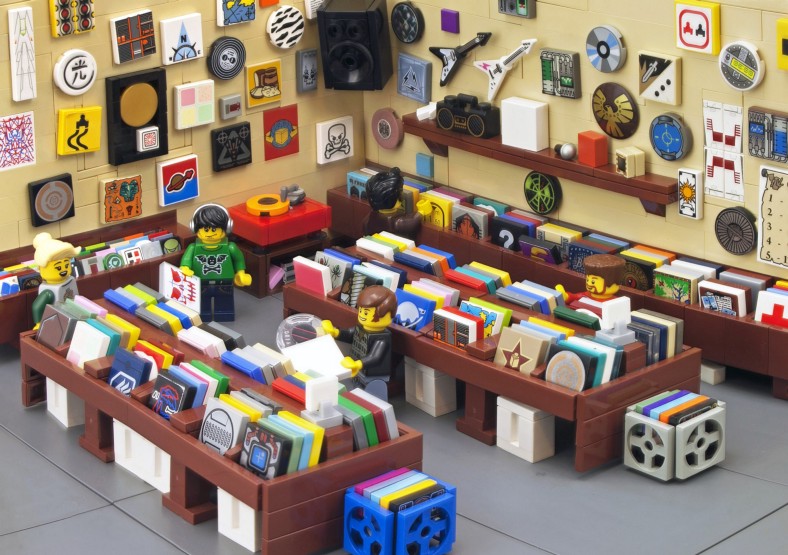
Image Source: Ryan Howerter on Unsplash
Finding the right content to upskill your internal team can be difficult. Despite the rapid proliferation of training portals and Massive Open Online Courses (MOOCs), sometimes these curricula do not meet the specific needs of organizations. With so much great educational content available online, and so much of it often free, it might seem pointless to recreate it for your company.
“We need to design our own curriculum by pulling together content from multiple online sources,” says Wendy Zhang, director of data governance and data strategy at Sallie Mae . A training plan should be based on the team’s background, role, and what is needed for success. Such an approach offers a win-win. That means you can reuse valuable online content while avoiding the limitations of a cookie-cutter approach.
In order to motivate the team to improve their skills, it would be a good idea to add a sense of game to training. When Zhang worked for an American financial services firm, he held fun contests to help his team develop new skills. The simple reward of a lunch with executives fostered a healthy sense of competition among team members and enabled fast-paced learning.
3. Bridge your team’s technical skills and domain expertise
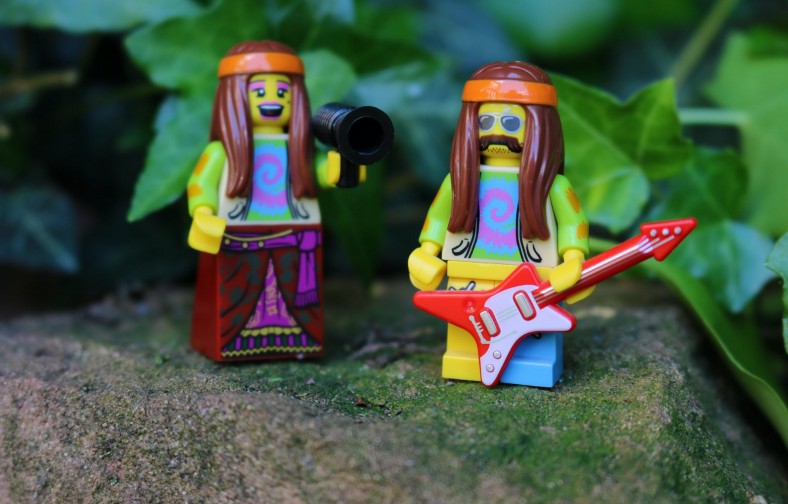
Image Source: Bob Coyne on Unsplash
Good AI solutions require the right mix of domain knowledge and technical expertise . People who have experienced upskilling often have a narrower perspective. Technical training often does not touch business applications, while business orientation may not be based on technology.
Fidelity Investments ‘ online analytics academy helps employees with business and technical backgrounds develop skills in artificial intelligence , big data and analytics. Todd James, SVP of Intelligent Automation at the company, said: “When we started working on AI, it became clear that we needed to bridge the AI perception gap between the data science team and the business team.”
“To address this challenge, we created Learning Days, an agile routine in which data scientists educate business teams with practical examples on identifying AI use cases. and provided a platform for sharing how best to work with the data scientist team, while the data science team received similar briefings on strategy, products and business processes from business partners added James. Learning Days closed the AI awareness gap and led to higher quality ideas and project implementation.
- Social science: Social science , which considers human social activities, is useful for inferring customer behavioral tendencies from data collected from customers .
- Data Science: Data science provides methods to analyze the data collected .
- Management science: In order to incorporate the results of data science projects into corporate activities, it is necessary to change the workflow and organizational structure . The science of management can help us to carry out these changes.
4. Allow experimentation and learning at work
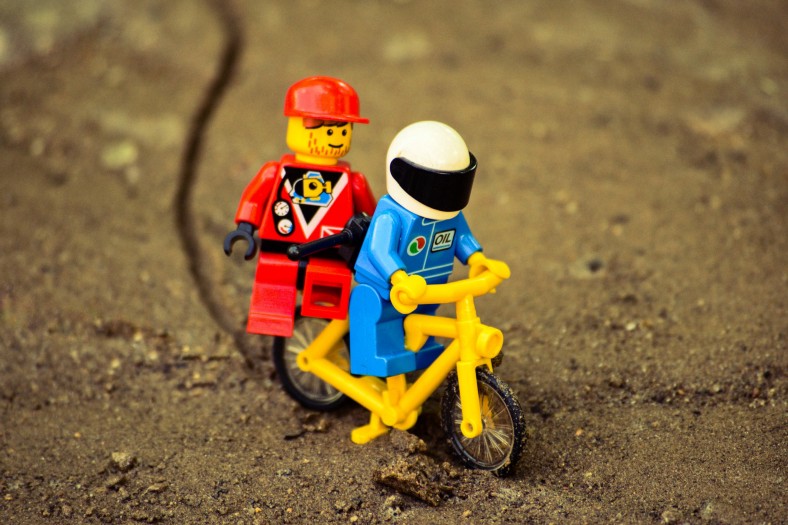
Image Source: Tolga Ulkan on Unsplash
In the words of Julius Caesar, experience is the best teacher. No matter how new the technology, it becomes flesh only after putting it into practice. No matter how good a course or training method is, it doesn’t matter if you don’t let your team experiment, make mistakes, and learn on the job.
“We are big believers in on-the-job training,” said Michael Cavaretta, senior manager of manufacturing analytics at Ford Motor Company . “Our team is made up of members with a variety of backgrounds, from industrial engineering to computer science. They rarely come,” Cavaretta said.
If internal candidates have a growth mindset and aptitude for learning, OJT can be designed. Designing OJT requires a mix of novices and experienced employees and clearly sets expectations during the shadowing period. “We define tasks that beginners can immediately tackle during the shadowing period so that they can apply what they have learned from those tasks. We create step-by-step tasks that need to be done,” Palmer said of OJT.
Shadowing in this article is used in the sense that the trainee immediately repeats the example shown by the trainer in OJT, instead of its original meaning.
Skill balance in the team
Training with a methodology and its application will enable teams to upskill, and the four approaches described above will hone the competencies necessary for AI work. And by creating an environment that nurtures skills, we must strike a balance between AI competencies and soft skills such as curiosity, creativity, and communication. When you do these things, your team will be ready to build data science solutions that are not just interesting, but have business impact .